AtScale and Databricks a Match Made in Heaven
How does a successful implementation of Data Analytics strategy help organizations?
AtScale Business metrics stay consistent across the organization. Analysts can access a broader range of data.Promotes self-service with business-friendly semantics.
- Insights in more easily disseminated through preferred BI tools.
- Security, compliance, and governance policies are enforced.
Once we have a successful Analytics strategy in place within the Organization we can start scaling the platform for our AI/ML needs providing a powerful platform for data teams to build robust business analytics programs — making analysts and data scientists more productive and decreasing time to insight should be next on our roadmap.
Gartner Magician Databricks with its revolutionary Lakehouse architecture pattern that brings Data Lake and Datawarehouse platform together to support enterprise Data Science and Analytics programs is adopted by many organizations when they start on their journey towards digital transformations. One of the features of Databricks to centralize disparate data sets in a highly scalable, cloud-based infrastructure is the foundation for democratizing data across organizations.
An analytics semantic layer that establishes a single view of critical business metrics and common analytics vocabulary across all data consumers solution bridging business intelligence and data science teams through a clean representation of key business metrics and important analysis dimensions that ensures consistency across all users even if underlying data sources change. The end result teams spend more time delivering insights, and less time manipulating and prepping data. This is achieved by establishing an integration layer within the enterprise data fabric.
AtScale process
- Before you proceed further it is recommended that you follow my first article on the benefits of a Semantic layer to get familiarized with the terminologies that will be used in the article below
- AtScale delivers a business-oriented semantic layer sitting on top of Databricks that provides live, high-performance query access to data stored in a Databricks Lakehouse while forming a single source of governed analytics for all data consumers to leverage. It accelerates end-to-end query performance while pushing down the compute to Databricks clusters.
- The AtScale No Code modeling approach helps build sophisticated models across disparate data sources with no SQL and rapidly iterate models that can be shared across analysis teams.
- AtScale ability to move and manipulate data with Python scripts helps data scientists to move data from AtScale into their models or AutoML platfoms — simplifying feature engineering and supporting consistency for production models. With the supports to write-back model results through the semantic layer the BI teams can publish model results to analysts and managers using existing dashboard and reporting tools
- AtScale Security and Governance extends role-based security and governance policies of source data to analytics consumption.
- AtScale enhances analysis with external data sources by blending enterprise data with third-party cloud data services within the AtScale model.
Benefits of AtScale and Databricks together
- Eliminate data movement as there is no need to create a separate query layer such as Datawarehouse/DataMart or cubing solution just for the kind of performance you need. No data movement means there is no copies of data and no consumer gets to see stale data or subset of data because all of the data stays in the Lakehouse architecture which we have heavily invested in.
- Modernize legacy “Cube” architectures like SSAS to support a solution that enables blazing fast dimensional analysis by creating aggregates on Databricks.
- Delivering a “Diamond Layer” for analysis ready data across popular BI, Data Science, and ML services with a semantic layer that enable customers the ability to visually model data providing self-service analytics to users within organization.
- Extending tooling support through dialect support for DAX, MDX, Python, and SQL which makes is easier to integrate with tools like Power BI and Excel internally AtScale uses Data Virtualization to convert above queries to Databricks SQL.
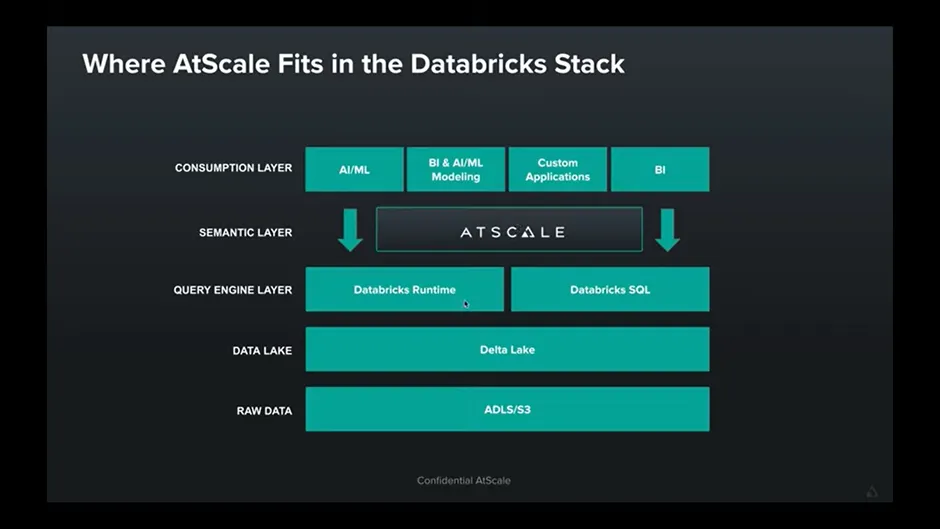
System Design before and After AtScale
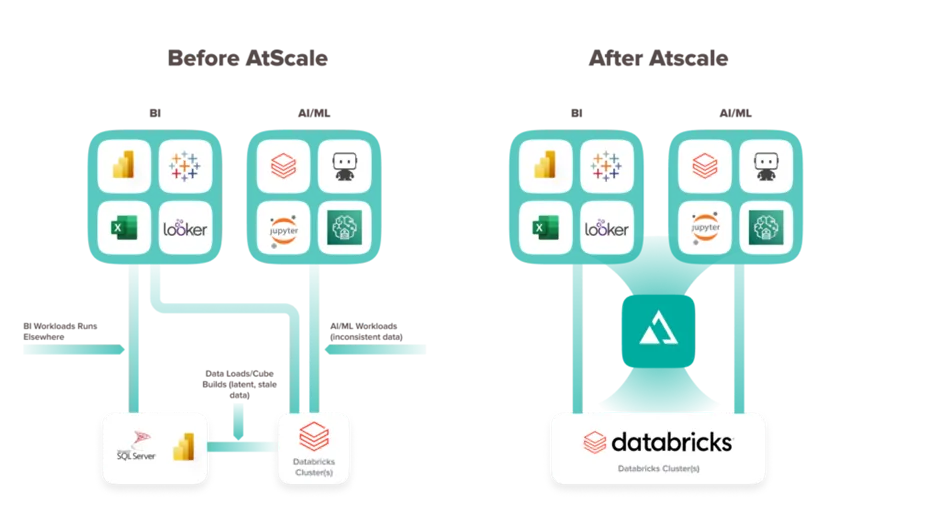
An Architects view of reference architecture for implementation
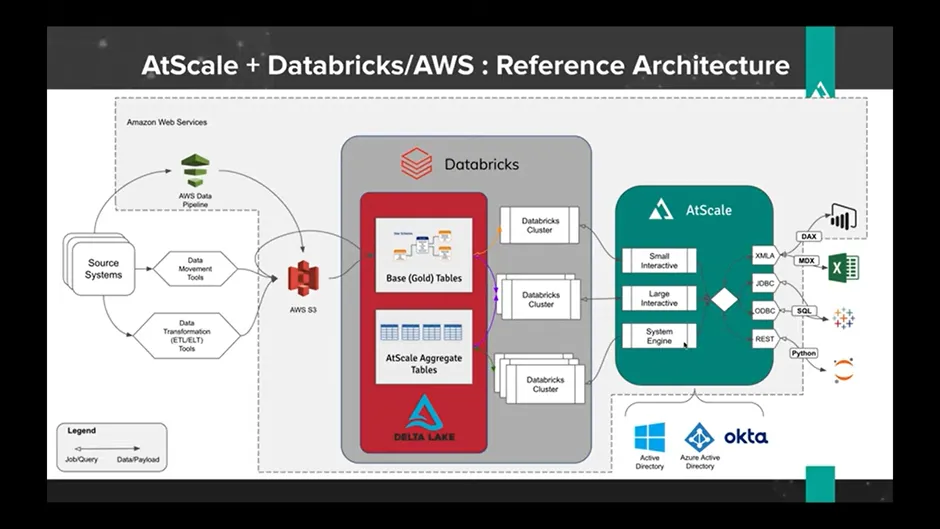